On April 6th 2023 we implemented a major update to the Cibo Labs PastureKey Service, and the Australian Feedbase Monitor.
Over the last 12 months we have been working closely with our clients and collaborators to improve our total standing dry matter (TSDM) predictions in priority areas where model performance was not meeting expectations, largely due to a lack of data describing specific pasture systems and unprecedented seasonal conditions. These priority areas included:
- The southern rangelands of WA, SA and NSW;
- Pasture under mulga and brigalow systems in western and central QLD
- High biomass, senesced temperate fescue and Phalaris.
The most significant challenge was collecting data to predict senesced high-biomass temperate pastures in eastern NSW where vast areas had spring, summer and autumn growth accumulated across many under-stocked farms. Throughout 2022, the Cibo Labs team and many collaborators collected over 1000 additional sites to capture the biomass and phenology of these pasture systems which often exceeded 6,000kg/ha.
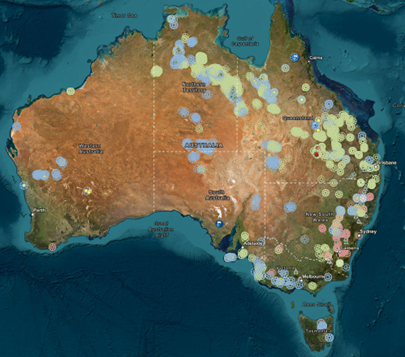
![]()
|
![]()
|
Figure 2. A Tall Fescue pasture estimated from quadrats cuts to be 10,000kg/ha. |
Figure 3. An example of the location of field transects and quadrat data collected using our Biomass Collector App (Survey123). |
![]()
|
Figure 4. Pasture biomass model predictions compared to field sites using 10-fold cross validation. Points coloured by the density of nearby points highlighting areas with many points overlaying each other. The plot also includes the estimated error of field observations and prediction confidence envelope. |
For 5100 field sites we used random sampling of 60% of the data to develop the model, 30% of the data to provide internal validation, and 10% to test the model. Outliers can be due to poor model performance, rapid growth due to rain events close to the sampling, or pasture utilisation after the field sampling has taken place. It is important to note that the field data has an overall Mean Absolute Percentage Error (MAPE) of 40%. The lowest MAPE is from the pasture cuts, however these are also highly variable with a MAPE of ~30%.
The Median Absolute Error (MAE) of the model increases when a larger range of TSDM observations is considered. When TSDM values of up to 2000 kg/ha are considered MAE is 204 kg/ha and the MAPE is 19%. Importantly, the model error matches the error in the field data up to 2000kg/ha.
The integration of the northern and southern models into a seamless national biomass prediction model has been a significant success and to our knowledge the only commercial implementation of such a model anywhere in the world.
The “living model” approach to the national biomass prediction service is now well established within operational systems. Our ability to reliably estimate pasture biomass is only as good as the data collected to train the models and our ability to adequately represent the pasture types and conditions important to grazing management decisions. This has been extremely challenging to achieve with highly variable seasonal weather and pasture conditions since development began in 2018.
Our focus now is to continue to work closely with individual producers to identify regions, pasture types and seasonal conditions where model performance needs to be improved and to coordinate data collection.
The freely available Biomass Collector App provides a capability for nationally consistent data to be easily collected and fully utilised by the industry and research organisations.
The significant improvement in model performance does however, also create a challenge with comparing time-series data. Any data generated within the Australian Feedbase Monitor (AFM) or the PastureKey service prior to April 6, 2023 will be using the prior model, and is likely to under-estimate high biomass senesced pastured, and should not be used for any time-series analysis. If you wish to look at inter-seasonal comparisons you should use the coarser AFM data which is less sensitive to paddock-scale variability. Cibo Labs can also back-process the data with the new model on request.